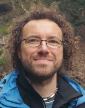
Biography
Biography: Marco Dominietto
Abstract
Cancer is a multifactorial and heterogeneous disease. The corresponding complexity appears at multiple levels, from molecular to physiological behavior, and determine the different evolution of the same disease in different patients or the different response to the same therapy. Such complexity can be characterized by a set of quantitative phenotypic observables recorded in time-space resolved dimensions by using multimodal imaging approaches. These observables, called here features, measure single variables that account for single physiological processes both in tumor and hosting tissue, e.g. tumor oxygenation, vascular permeability, glucose consumption, etc. Clearly, in tumor progression all these features interact together and determine its evolution. The integration of all these information in a comprehensive framework is therefore one of the key to understand cancer disease in its entirety. Complex networks offer in this regard an ideal solution for the integration of multiple information layers at different space and time scales. Single parts of the tumor that correspond to voxel volumes on imaging readouts, constitute the nodes of the network that are linked together on the basis of the features distributions. Statistical inference on topological properties and dynamic evolution of the network allows the determination of the stage of the tumor and predicts its evolution. Moreover, treatment as chemotherapy or radiotherapy can be accounted as perturbation of the network. The advantages of this approach are in terms of a) integration of several features in a single framework, b) estimation of the interaction between tumor and hosting tissue and c) prediction of treatment efficacy.